In Parts I (Solving the Overlap Query Problem in PostgreSQL) and II (Overlapping Ranges in Subsets in PostgreSQL) of this series, we used the GiST index type – and its lesser known cousin: SP-GiST – to turbocharge the performance of overlap queries. But GiST indexes are extremely versatile, with uses far beyond the examples I have used so far.
In this final article, we’ll explore some of the many other ways they can be used.
Stuck in the Middle With You
We’ve used only one operator in all our queries so far: the “&&
” operator, which returns true if two ranges overlap to any degree. There are other range operators that GiST can use to accelerate query performance. The operators “<<
” and “>>
” determine whether one range is entirely to the left or the right of another. These aren’t terribly interesting, as an ordinary B-Tree index can easily support the same type of query.
More useful are the inclusion operators ‘<@
‘ and ‘ @>
‘. These tell you if the first range lies entirely inside the second or vice versa. To illustrate their use, consider our Part I example of museum patrons and security outages. (If you haven’t been following, along, the code to create these objects is in the Appendix of Part II)
Our original query identified patrons who were present during some portion of an outage (any amount of overlap). But to find patrons who were present for an entire outage (the outage both began and ended during their visit) we write this query:
1 2 3 |
SELECT * FROM patrons p CROSS JOIN outages o WHERE tsrange(p.enter,p.leave) @> tsrange(o.start_time,o.end_time); |
Conversely, to locate patrons who arrived and left during an outage (their visit lies entirely inside the outage), either reverse the order of operands in the WHERE clause or change the operator to point the other direction:
1 |
WHERE tsrange(p.enter,p.leave) <@ tsrange(o.start_time,o.end_time); |
It’s easy to remember these operators using the same rule taught in elementary school math: the little end of the “<
” and “>
” signs points to the smaller range.
These operators will work without any index being defined, but the query will be extremely slow. If we rewrite the query using standard ‘<'
and ‘>
‘ operators, an ordinary B-Tree index will provide some help:
1 |
WHERE p.enter < o.start_time AND p.leave > o.end_time |
But the performance gains from a GiST index can be astronomical. Running the above queries on our Part I/II test data yields the following results.
Scenario | Execution time |
Non-indexed |
423 sec. |
B-Tree index (alternate form query) |
20.1 sec. |
GiST format index |
0.6 sec. |
SP-GiST index |
0.2 sec. |
Reminder: our test data is well-suited to SP-GiST. Against data sets with more overlap, GiST may perform better.
The Query from Another Dimension
So far we’ve only used GiST with one-dimensional data: date and timestamp ranges. GiST becomes even more useful with two-dimensional queries. If you think to yourself, “I don’t have exotic queries like that: just ordinary dates and numbers”, well you’re likely to be surprised.
To explain, let’s create a new set of test data: a table tracking sales of some (unnamed) commodity. Our table has four columns: a customer_id
, the sale date, and the bid price and ask price.
1 2 3 4 5 6 |
CREATE TABLE sales ( customer_id INT NOT NULL, sdate DATE NOT NULL, bid NUMERIC(9,2) NOT NULL, ask NUMERIC(9,2) NOT NULL ); |
Note: if you want to test this code on your own computer, there is code to generate a set of data in the appendix of this article, in the section of code named: — Script to test bid/ask queries. The data is randomized, so your output will not match exactly, but should be very close in usage.
Let’s assume we want to find sales where the bid price lies in a certain range and the sale price lies in another:
1 2 3 4 |
SELECT * FROM sales WHERE bid BETWEEN 10.00 and 12.00 AND ask BETWEEN 10.50 and 13.75 |
This is a two-dimensional query; the value range for bid price is one dimension, while ask price is the other. To better visualize this, let’s first examine a basic 2-D geographic query, the type GiST is commonly used for: finding a set of points that lie within a ‘box” of latitude/longitude values.
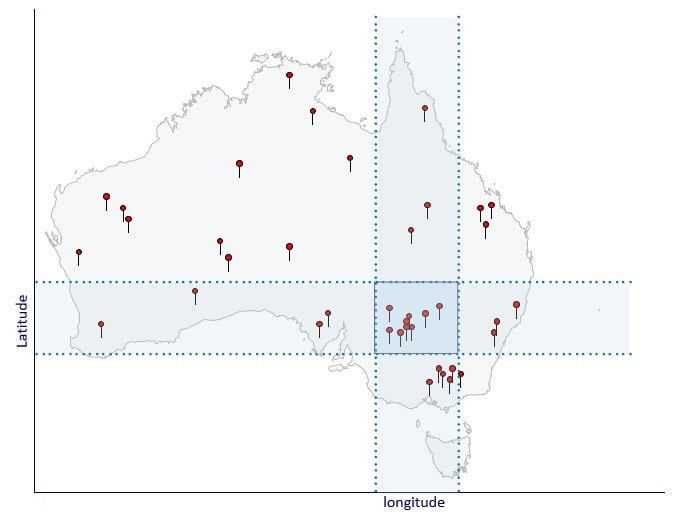
B-Tree indexes perform badly here. They can quickly locate one range – either the latitude or the longitude band show above – but then they’re forced to scan for the intersection with the other band.
Now let’s translate this concept to our commodity sales query. Just like our geographic query, we have two “bands” of values our query needs to intersect, bid price and ask price:
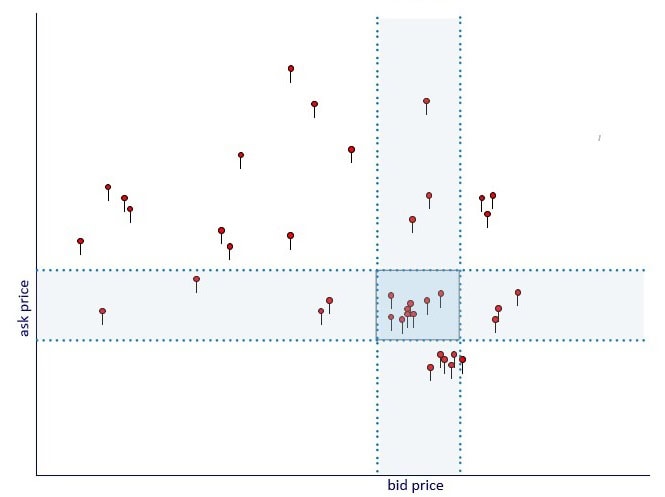
The intersection “box” isn’t a geographic region on a map, but it still lies within a two-dimensional space. And GiST absolutely loves queries like this. B-Trees use a one-dimensional structure, but GiST uses a two-dimensional analogue known as an R-Tree (SP-Gist uses a similar quadtree).
Let’s rewrite our bid/ask price query to use GiST. Since we’re searching for two-dimensional points, we create an index using a POINT
object, with bid price as one coordinate, and ask price the other:
1 |
CREATE INDEX ON sales USING gist(point(bid, ask)); |
To perform the actual search, we match this point to our desired search “box”. PostgreSQL’s default box()
function only accepts points though. To simplify the syntax, we define a box() function that accepts four numeric values:
1 2 3 |
CREATE FUNCTION box(int,int,int,int) RETURNS box -- define points as a box that includes bid and ask price RETURN box(point($1,$2),point($3,$4)); |
Now we can write our search query. The “<@
” inclusion operator finds points within a box. This query finds all rows with bid price between $600 and $620 and ask price between $615 and $625:
1 2 3 |
SELECT * FROM sales WHERE point(bid,ask) <@ box(600,615,620,625); |
OK, it works – but are the results worth dealing with this opaque syntax? How much faster is it than the traditional BETWEEN
syntax and an ordinary B-Tree index? Let’s run some timings! (The queries and indexes used for these tests are included in the Appendix)
The table below shows benchmark results for the various index types. Three different query parameter scenarios are tested, all returning the same number of rows. In the balanced scenario, the range of values for both search terms (bid and ask price) are equal. This corresponds to the horizontal and vertical search bands in the above image being equal width – each range by itself matches 0.3% of the table’s 50M rows.
The “favored” scenario divides the range of bid values by a factor of six, while multiplying the ask range by the same amount. This selects the same amount of data, but now the bid price is doing nearly all the work of filtering. If the table contains a B-Tree index starting with bid price, this is the best-case scenario. Similarly, the “adverse” query reverses this, widening bid price range by 6X and narrowing ask price similarly.
Query Performance (50 million rows) | |||
Query Parameters | |||
Index Type |
Balanced |
B-Tree favored |
B-Tree adverse |
No index |
4501 ms |
4501 ms |
4501 ms |
B-Tree (bid price only) |
10090 ms |
2430 ms |
4501 ms |
B-Tree (both bid & ask) |
75 ms |
59 ms |
201 ms |
GiST |
51 ms |
60 ms |
59 ms |
SP-GiST |
50 ms |
62 ms |
61 ms |
There’s a lot of information to unpack here. With no index, all three queries have identical (terrible) performance. An index on just one search term (bid price) doesn’t help much: the “favored” scenario sees a slight speed boost, but the adverse query fails to use the index entirely, while the balanced scenario actually slows down. Ouch.
A compound index on both bid and ask does much better performance-wise, but it is very sensitive to the search terms, with performance varying by more than a factor of 3 from the slowest to the fastest query. In the adverse scenario, the bid price range matches 900K of our 50M rows – compound B-Tree indexes perform poorly when their primary term isn’t very selective.
Our GiST index shines here. It ties the B-Tree in the favored scenario, is 50% faster on balanced queries, and a full 3.4X faster on the “adverse” scenario. More importantly, the performance of the GiST index is much more consistent. Between the favored to the adverse scenario the specificity of the search values changes by a factor of 36, yet the query time barely changes. This is the predictable behavior we want from an index.
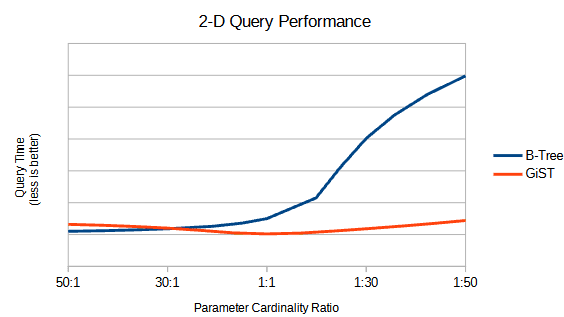
SP-GiST is also consistent on performance, but offers no advantage over basic GiST, and in fact is slightly slower in two of three scenarios. But hold tight: SP-GiST has an advantage that we’ll see below.
We could improve worst-case B-Tree performance by creating a second index, reversing the column order to “ask, bid”. But maintaining two indexes requires double the cost and storage and performance still wouldn’t match our single GiST index. And as the table increases in size, the B-Tree index will fall further behind.
Like an ordinary index, a two-dimensional GiST index can be made compound by adding additional columns. For instance, if we our bid/ask query needs to search for a specific commodity code, we can include this column in our index (note that this column does not exist in this table and is just an example. This type of index requires the btree-gist extension mentioned in Part II):
1 |
CREATE INDEX ON sales USING gist(point(bid, ask)<strong>, ccode</strong>); |
What if our query has more than two range parameters? Three ranges would turn our search box into a 3-D cube; four ranges a 4-D hypercube, etc, etc. Extending our 2-D approach to higher dimension indexes is possible, but beyond the scope of this article.
Luckily this is rarely necessary. A 2-D index on the two ‘best’ parameters (those with the highest cardinality) usually winnows the result set enough that a simple scan on the remaining criteria is fast enough.
A Warm Fuzzy Search
If the above results don’t convince you there’s value in a 2-D GiST query, let’s look at another type of query they accelerate … and by an even larger margin. This is the so-called kNN “nearest neighbor” search. Queries like this allow us to ‘fuzzily’ match multiple search criteria, returning the closest values found.
Using our sales table example, let’s assume we want the best match to a bid price of $570 and an ask price of $575. Either price can vary from our desired value; what we want are rows that have the least difference in both values simultaneously.
Writing this in standard SQL is messy, but PostgreSQL has a built-in operator to find the distance between points, the “<->” operator. Using the point() syntax from above, we can find closest matches by ordering off the results of this operator. Then we simply limit the query results to the number of “fuzzy” matches desired. For instance, the “LIMIT 1
” in this query finds the single best match:
1 2 3 4 |
SELECT * FROM sales ORDER BY point(bid,ask) <-> point(570,575) LIMIT 1 |
For the best “n” matches, increase the LIMIT
accordingly. Ordering the table in reverse (descending order) would give you the worst matches, though uses for this are rare (searches on an “opposites attract” dating site, perhaps?)
Queries like this don’t specifically require a GiST index, but performance will be abysmal for all but very small data sets:
Nearest-Neighbor Search ( 50 million rows, 50 closest matches) | |
Index Type |
Performance |
None |
12981 ms. |
B-Tree |
12981 ms. (index unused) |
GiST |
10.1 ms. |
SP-GiST |
7.3 ms |
Here, SP-GiST outshines its bigger brother, turning in nearly 30% better performance than GiST, and well over 1000x faster than the un-utilized B-Tree index. That’s speed worth working for.
The “<->
” operator calculates “Euclidean” distance – using the Pythagorean theorem from junior high school – meaning that if both prices differ by $10, the distance becomes 14.1, not 20. It also weights both search criteria equally. If we wanted our fuzzy search to be more sensitive to differences in bid price than ask price, we could scale the value accordingly when creating the point object (though we need to perform the same scaling when creating the index.)
A Date With Destiny
Multidimensional queries aren’t limited to numeric values. With a little effort, we can include dates, even querying a date range in conjunction with a numeric one. Using our sample sales table, let’s try to write the following query to be usable by a 2-D GiST index:
1 2 3 4 |
SELECT * FROM sales WHERE sdate BETWEEN '01-01-2024' and '02-01-2024' AND bid BETWEEN 600 and 650 |
Again there are two independent ranges of values – one a date, the other numeric – that jointly comprise a 2-dimensional query. To use a GiST index with this, we need to be able to create point() and box() objects, but these functions require numeric arguments. The easiest way to do this is to subtract from each date an (arbitrary) fixed date, transforming the original value into the number of days elapsed. We could do this directly, but it will simplify the syntax if we create another helper function. The unimaginatively named “day_cnt()” returns the number of days since Jan 1, 2000:
1 2 |
CREATE FUNCTION day_cnt(date) RETURNS int RETURN $1 - '01-01-2000'; |
Now index on a point() object containing the sales date and bid price:
1 |
CREATE INDEX ON sales USING spgist( point( day_cnt(sdate), bid)); |
To use the index, pass the query dates to our helper function as part of constructing the query box:
1 2 |
SELECT * FROM sales WHERE point( day_cnt(sdate),bid) <@ box( day_cnt('1-1-2024'),600, day_cnt('02-01-2024'),650 ); |
This syntax is still convoluted, but if you use it often, you can define point() and box() helper functions that accept dates directly, making it just about as straightforward as a standard SQL query.
Performance for this query is similar to the bid/ask scenarios we ran above: tying a B-Tree index when the first parameter range is tiny compared to the second, and several times faster in the reverse case. And we can also perform fuzzy matches on dates. This query finds the three closest matches to a sale made Aug 10,2024 for $500:
1 2 3 4 5 |
SELECT * FROM sales ORDER BY point( day_cnt(sdate),bid ) <-> point( day_cnt('8-1-2024’),500 ) LIMIT 3; |
Reminder: the closest matches are based on date and price; both can vary at once. Because we’re converting sale date to a count of days, every $1 difference in bid price equates to 1 day difference in sale date, i.e. the two rows below are equally “far” from our search criteria:
sdate: 08-15-2024 bid price: $500 (14 day difference)
sdate: 08-01-2024 bid price: $514 ($14 difference)
If we modify day_cnt()
to return the number of weeks, then a $1 price difference equates to a sale date difference of one week. Alternately, if we divide bid price by 10 before indexing, everyday difference in sale date equates to a $10 price difference.
Conclusion
Even after three articles on the subject, we’ve just scratched the surface of what’s possible with flexible, multi-purpose GiST indexes. The next time you’re faced with a tough index strategy challenge, stop to consider if GiST may provide a solution.
Appendix I — test data script
1 2 3 4 5 6 7 8 9 10 11 12 13 14 15 16 17 18 19 20 21 22 23 24 25 26 27 28 29 30 31 32 33 34 35 36 37 38 39 40 41 42 43 44 45 46 47 48 49 50 51 52 53 54 55 56 57 58 59 60 61 62 63 64 65 66 67 68 |
-- ------------------------------------------------------------------- -- Script to test patron / outage overlap. Uses data from Parts I/II. -- -- B-Tree form query SELECT * FROM patrons p CROSS JOIN outages o WHERE p.enter < o.start_time AND p.leave > o.end_time -- GiST form query SELECT * FROM patrons p CROSS JOIN outages o WHERE tsrange(p.enter,p.leave) @> tsrange(o.start_time,o.end_time); -- ------------------------------------------------------------------- -- Script to test bid/ask queries. -- -- Create data CREATE TABLE sales ( customer_id INT NOT NULL, sdate DATE NOT NULL, bid NUMERIC(9,2) NOT NULL, ask NUMERIC(9,2) NOT NULL ); -- Generate 60M random records. This creates a statistical correlation between -- search terms, ensuring that ask price remains within +/- 200 of bid price INSERT INTO sales ( SELECT ROUND(RANDOM() * 99999), CURRENT_DATE - '1 DAY'::INTERVAL * ROUND(RANDOM() * 365), r, r-200+ROUND(RANDOM()*400) FROM (SELECT 100+ROUND(RANDOM()*6500) AS r FROM generate_series(1,60000000)) as RowSet ); -- to test with uncorrelated data, use this insert instead. -- this type of data should perform better with SP-GiST -- TRUNCATE TABLE sales; -- INSERT INTO sales ( -- SELECT ROUND(RANDOM() * 99999), CURRENT_DATE - '1 DAY'::INTERVAL * ROUND(RANDOM() * 365), 100+ROUND(RANDOM()*6500), 100+ROUND(RANDOM()*6500) FROM generate_series(1,60000000) ); -- Create BTree and GiST indexes. CREATE INDEX sales_btree ON sales USING btree(bid,ask); CREATE INDEX sales_gist ON sales USING gist( point(bid,ask)); -- To test SP-GiST performance, uncomment and run the following lines. Note that GiST -- and SP-Gist cannot be tested simultaneously. -- DROP INDEX IF EXISTS sales_gist -- CREATE INDEX sales_spgist ON sales USING spgist( point(bid,ask)); -- Add helper function for queries CREATE FUNCTION box(int,int,int,int) RETURNS box -- define points as a box that includes bid and ask price RETURN box(point($1,$2),point($3,$4)); -- -- TEST QUERIES -- -- BTree favored scenario: both search terms roughly equal cardinality. First -- query uses BTree index; second query GiST (or SP-GiST if that index exists) SELECT * FROM sales WHERE BID BETWEEN 600 AND 605 AND ask BETWEEN 550 AND 670 SELECT * FROM sales WHERE point(bid,ask) <@ box(600,550,605,670) -- balanced scenario EXPLAIN ANALYZE SELECT * FROM sales WHERE BID BETWEEN 600 AND 630 AND ask BETWEEN 610 AND 640 SELECT * FROM sales WHERE point(bid,ask) <@ box(600,610,630,640) -- B-Tree adverse scenario SELECT * FROM sales WHERE BID BETWEEN 600 AND 780 AND ask BETWEEN 610 AND 615 SELECT * FROM sales WHERE point(bid,ask) <@ box(600,610,780,615) |
Load comments